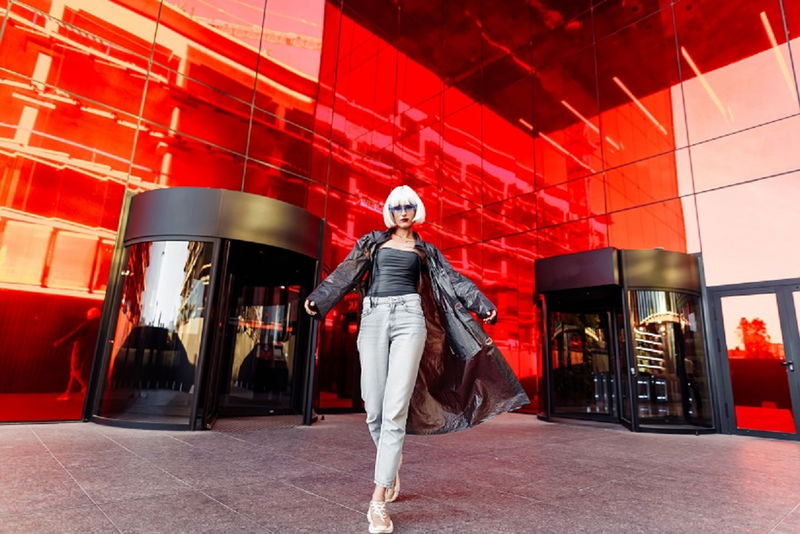
Real-time analytics refers to using data and resources immediately upon their entry to a system for analysis, offering users instantaneous computer responsiveness as soon as they come online. Real-time can often refer to streaming data structures or automated operational decisions made in real time via policy enforcement or robotic process automation systems, making real-time data analysis relevant in such instances.
Real-time data analytics examine and visualize real-time computer system data as it's displayed, unlike historical analysis, which requires batch analyses of past information. Data scientists can utilize real-time insights analytics for various uses:
- Resolve operational decisions and implement them consistently into production activities such as business transactions and processes.
- Real-time dashboard displays with continuously updating transactional data sets rapidly emerge as industry-standard solutions.
- Utilizing current predictive and prescriptive analytics.
- Present facts, both current and historical, simultaneously.
Real-Time Analytics Systems Software Essential Components
- An aggregator that gathers data event streams -- and perhaps batch files -- from a variety of data sources
- A broker that makes data available for consumption
- An analytics engine that analyzes the data, correlates values and blends streams together.
The stream processor is the system that receives and sends data streams and executes the application and real-time analytics logic.
How Real-Time Analytics Works?
Real-time analytics ensure that data analysis occurs near its source as much as possible; real-time analysis often occurs near network edges. Aside from edge computing, other technologies aid real-time analysis:
- Processing in memory (PIM) refers to an innovative chip architecture in which the processor is integrated within a memory chip to reduce latency.
- In-database analytics (IDA) is a technology that enables data processing within databases by embedding analytical logic directly within them.
- Data warehouse appliances are combinations of hardware and software products designed specifically to support analytical processing. An appliance allows a purchaser to deploy high-performance data warehouses instantly out of the box.
- In-memory analytics refers to querying data that exists solely within random access memory instead of physical disk storage devices.
- Massively parallel programming (MPP) refers to the coordination of parallel processing by multiple processors working on individual portions of a program using their respective operating systems and memories.
Real-time analytics apps should offer low response times and high availability to ensure valuable real-time streaming data collection. They must also handle large terabytes of information without breaking down while responding promptly to inquiries within seconds.
Real-time analytics applications should also be utilized to manage dynamic data sources that arise as business and market conditions change; such information must be addressed immediately for optimal efficiency.
Real-time big data analytics offers many advantages:
- Increase business returns while simultaneously decreasing operational expenses
- Introduce an era where machines interact through the Internet of Things using real-time information to make autonomous decisions about themselves.
An increasing volume and variety of data require different solutions, each catering to specific appliances like software and hardware systems; some other technologies use particular combinations like CPU/memory chips/databases with analytics features to meet this need.
Benefits Of Real-Time Analytics
Thanks to real-time analytics architecture, businesses can instantly respond, identify user behavioral trends more rapidly, seize opportunities that might otherwise go undetected, and prevent issues before they arise.
Real-time analytics platforms help companies significantly lower risk across their operations. Unlike historical analytics, which relies on assumptions derived from past scans or incidents, real-time systems analytics uses actual data to predict outcomes and offer alternatives - providing us with a window into current situations.
Real-time analytics also offers other advantages:
- See Events: Real-time data processing visualization allows businesses to see events as they happen throughout their organization; historical information must only be put in a chart as a snapshot.
- Increases competitiveness: Companies using real-time analytics can detect trends and benchmarks faster than their rivals, who rely solely on historical data for this task. Furthermore, real-time analysis allows firms to evaluate partners and competitors instantly as performance reports come through.
- Precise Information: Real-time analytics emphasizes instant studies that consistently contribute towards focused outcomes, saving both time and resources by eliminating data collection that does not contribute any tangible benefit.
- Cost Saving: Real-time technologies may appear costly initially, but their long-term benefits make them worthwhile investments. They allow resources or information to be utilized more quickly without delays arising, further helping businesses save costs on delays caused by traditional systems.
- Faster results: Classifying raw data instantly allows queries to collect relevant information more efficiently, leading to speedier trend prediction and decision-making processes.
Read More: Worth $80K? Real-Time Reporting And Analytics In CRM ERP Software?
Real-Time Vs. Traditional Analytics
Real-time analytics make real-time processing of large volumes of data possible through massively parallel programming, while traditional batch analytics offer scheduled processing and cost efficiency but cannot provide timely insights and respond promptly to real-time streams of information.
Traditional analytics provide business customer experience insight, storing and indexing small amounts of historical data, which they can access at will. When performing routine tasks like compiling paychecks or producing sales reports, batch processing may be used instead.
Real-time analytics use cases provide instant insights for tasks requiring immediate analysis. Examples may include:
- Monitor for patient safety
- detect fraud
- respond in an emergency situation
Real-Time Analytics Examples
Real-time analytics example include, but are not limited to:
- Financial organizations can now perform instant credit evaluations. Instant updates on each person's credit score allow financial organizations to quickly make decisions regarding extending credit to customer behaviors instantly.
- Financial trading decision-making is informed by real-time monitoring analytics of significant data sources like financial databases, social media networks and satellite weather stations used by institutions as immediate tools for informing their purchasing and selling decisions.
- They are focusing on advertising. To increase their chances of sale, business processes can utilize real-time analytics to offer incentives and promotions directly in-store while customers browse merchandise.
- Smartwatches and wearable technology use real-time analytics that have proven lifesaving by monitoring metrics like heart rate.
- Services for Emergencies and Humanitarian Needs. Incident responders can benefit from harnessing powerful information sources like traffic, weather and geospatial data to make more informed and effective decisions that increase their capacity to respond to emergencies or events by connecting real-time analytical engines directly to edge devices like drones.
What's The Future Of Real-Time Analytics?
Real-time analytics will exponentially affect pharmaceutical marketing and sales shortly. Instead of solely using conventional techniques for consumer behavior analysis and market trends analysis, more pharmaceutical companies may begin adopting real-time analytics technology as part of their marketing strategies to gain a deeper understanding of consumer preferences while simultaneously optimizing marketing to increase both sales and profit while decreasing expenses through precise projections.
Real-time analytics are also revolutionizing higher education. Business decisions can start advertising to potential students who, according to factors like exam results, academic status and finances, are most suited for their school. Real-time predictive analytics also assist educational institutions with predicting debt burden and earnings post-graduation and helping predict which students may graduate and use their degree successfully after their studies.
Unfortunately, due to an ever-increasing machine and technological device count and increasing data accumulation rate, extracting meaningful insights from this mass of information becomes increasingly challenging. One solution may be Elastic stack - an open-source collection of tools that centralize, store, process and show log data in real-time decisions from various machines or log sources in real-time - thus offering one way to meet this challenge. Computer programs are seen as having an open-source future - especially those targeted towards data-driven fields like business intelligence.
Want More Information About Our Services? Talk to Our Consultants!
Conclusion
Real-time streaming analytics allow business user behavior of all sectors to realize additional value from their data quickly. By protecting networks from security threats and optimizing inventory modeling techniques, streaming analytics give enterprises access to better decisions more confidently and accurately than before.
Real-time analytics has transformed businesses' operations by offering insightful data that supports swift decision-making. By employing essential technologies like in-memory computing, streaming data processing time, machine learning, and artificial intelligence, businesses can use their data to outshout rivals and spur expansion. Companies must prioritize data infrastructure integration scalability while facing the challenges associated with real-time analytics deployment.