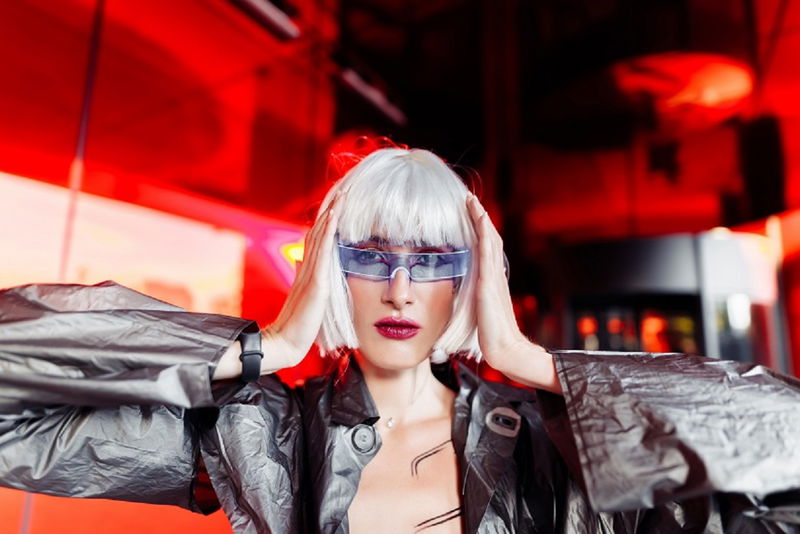
Digital transformation is the main engine powering the business world of today. For organizations to benefit from the technical breakthroughs of Industry 4.0, they are crucial. Machine learning and artificial intelligence (AI) technologies can be employed in real-time. They can automate activities thanks to enterprise services that are dependable and efficient. This has a significant effect on the digital transition. Enterprise resource planning (ERP) application improvement is one of ML's most important effects. Let's start by discussing what ERP is and how machine learning may help with its growth.
The business world of today is being heavily influenced by digital transformation. Companies that wish to benefit the most from technological advancements in Industry 4.0 will require them. The application of machine learning and artificial intelligence technologies in real-time and the automation of processes are made feasible by enterprise services that are effective and error-free. This has a big impact on how technology is changing.
The way that the modern ERP system manages business operations and data must alter. The ERP system has not changed. Over time, they have undergone significant modification. Many improvements have been made to ERP programmes to increase their efficiency, including capabilities for process automation, data automation, reminders, alerts, visualization, and data representation.
It is now entirely accessible online and has an interactive interface that is simple to use. The way we approach enterprise resource planning is evolving as a result of the technological revolution. Fast innovation has enhanced the application and provided a new layer to the ERP system. Algorithms for machine learning and artificial intelligence (AI) are available to assist. These words are frequently used in the terminology used today. AI can change everything and has already affected almost every global industry. The potential improvement of enterprise resource planning (ERP) software is one of the main effects of ML. Let's first define ERP and examine how machine learning can aid in the creation of ERP.
Enterprise Resource Planning: What is it?
Enterprise resource planning is known as ERP. Planning and managing virtual supply networks, financial operations, and production are made easier with ERP software and programmes. These modules are offered by many different systems. ERP software has a wide range of functionalities and business models. To automate and streamline company processes, there are a variety of enterprise resource planning technologies available. Enterprise resource planning (ERP) is a platform used by businesses to manage and connect the most crucial areas of their operations. ERP software programmes are vital for businesses because they let them adopt resource planning and combine all of the activities necessary to manage their operations through a single system.
ERP software systems such as enterprise resource planning (ERP) aid businesses in streamlining essential operations and enhancing productivity. Enterprise resource planning (ERP) software coordinates data flow between corporate activities to streamline enterprise operations. With a single platform, it may connect a business's finances, supply chain, procedures, and commerce, as well as its manufacturing and human resource operations.
Siloed systems are unable to sustain future expansion or be expanded outside everyday business activity. However, the majority of businesses have operational and financial systems. Designs need to be able to adapt as companies develop and their needs change. This article will define ERP and describe how it can increase your company's productivity and adaptability.
Describe Machine Learning
AI includes a subset called machine learning. Without the need for programming, machine learning allows devices (mainly computers) to learn from the past and data. Machines will be able to make decisions about the future using data and occurrences from the past. It could be challenging to comprehend the complex nuances of artificial intelligence (AI) or machine learning (ML). We can all agree that these notions and terminologies will predominate in the future. The way we approach and view a problem has been completely transformed by AI.
Even though they are not explicitly programmed, software applications may now predict events more accurately because of machine learning (ML), a subset of artificial intelligence (AI). Machine learning algorithms forecast future output values using historical data. The rapidly expanding area of data science includes machine learning as a fundamental component. To create predictions or classify data, algorithms can be trained using statistical techniques. This can help data mining initiatives unearth important insights. Business decisions are guided by these insights, which also help to improve crucial growth indicators. As big data evolves and grows, there will be a greater need for data scientists. To locate and supply data for the most important business questions, data scientists will be required.
The Importance of Machine Learning
Why is machine learning used? A growing amount and variety of data, affordable and easy access to computing power, and high-speed Internet are all contributing to the importance of machine learning. This aspect of digital transformation enables the quick and simple creation of models capable of performing accurate and timely analysis on big, complicated data sets.
Machine learning can be applied in a variety of ways to lower expenses, enhance the quality of life, and manage risks. Additionally, it can be used to detect cybersecurity breaches, enable self-driving cars, and promote goods and services. As we have more access to data and computing capacity and are likely to integrate machine learning into many facets of our daily life, it is becoming more and more prevalent. Since the beginning, machine learning has existed. The phrase "machine learning" was first used by Arthur Samuel, an IBM computer scientist and pioneer of AI and computer games. Samuel wrote a checkers-playing computer programme. To forecast future events, the programme used algorithms and gained additional knowledge through play.
Read More: Machine Learning And Deep Learning Are Becoming Increasingly Important For Businesses
Use of Machine Learning
A basic application of ML that we've used for years is spam screening in emails. Multinomial Naive Bayes Classifier is a simple spam filter. With this machine learning method, you can distinguish between spam and non-spam terms. After then, you decide whether new sentences sent to you via email meet your criteria for spam by comparing the frequency of spam terms that appear in penalties to the frequency of spam words that you have not deemed to be spam. Online marketers utilize comparable ML approaches to deliver adverts based on a user's online activity. Automation of routine chores, rule-based processes, and predictive analysis are all made easier by machine learning (ML).
Machine learning is essential because it enables businesses to identify patterns in corporate operations, customer behavior trends, and the development of new goods. Many of the most successful organizations in the world today, such as Uber, Google, and Facebook, rely heavily on machine learning. Machine learning has become a competitive edge for many businesses.
Types of Machine Learning
Machine learning algorithms can be trained in a variety of ways, each with advantages and disadvantages. Machine learning can be roughly categorized into the following primary types thanks to these techniques and learning strategies:
Monitoring Machine Learning
Supervised machine learning is one of the most used machine learning techniques. Because the algorithm learns by utilizing a collection of training datasets, this process is known as supervised learning. It is comparable to a teacher instructing his pupils. When the algorithm reaches an appropriate threshold for reliable performance, it stops learning. Data scientists train algorithms using labeled data and offer the variables they want the algorithm to look for correlations between. The algorithm's input and output are defined.
Supervision is required for this kind of machine learning (ML). Machines can anticipate outputs using training that they receive from labeled data after being trained. Some input and output parameters have been mapped according to a labeled dataset. The machine is assigned the input and output parameters. The device is introduced using the test data so that it can forecast the result.
Automatic Machine Learning
Unsupervised learning is a less well-known type of machine learning in which there are no output variables, only input data. Unsupervised learning is a type of machine learning that, in contrast to supervised learning, does not produce accurate results. To learn more, this kind of machine learning looks into the underlying structure of the data. Automated algorithms can be used to discover the current and upcoming designs in data. Algorithms used in this sort of machine learning are those that have been learned on unlabeled data.
The algorithm seeks out relationships between different data sets. To train algorithms, predetermined data is used. They also make predefined predictions and recommendations. Unsupervised learning is a type of instruction where supervision is not necessary. The system can predict the outcome without care after being trained with an unlabeled dataset. Unsupervised learning methods seek to classify the unsorted dataset based on patterns, similarities, and differences.
Semi-supervised Learning
Both of the methods mentioned above can be used in conjunction with machine learning. An algorithm may be fed primarily with training data by data scientists. Still, the model can explore the data and develop its understanding.
The best aspects of supervised and unsupervised machine learning are combined in semi-supervised knowledge. Semi-supervised education trains its algorithms using both labeled and unlabeled data. Both datasets are used in semi-supervised learning. Several of the primary drawbacks are addressed by this. Let's use a college student as an example. In college, supervised learning refers to the process of a student acquiring a topic while being guided by a teacher. Unsupervised learning is when a student studies the same subject on their own at home, unsupervised by a teacher. When a student reviews the material while being guided by a college instructor, this is known as semi-supervised learning.
Reinforcement learning
To train a machine to adhere to a set of precise rules, data scientists frequently utilize reinforcement learning. Data scientists can construct an algorithm to finish a task and then provide it with cues that are either positively or negatively conditioned to assist it. Most of the time, the algorithm will choose the next course of action. Feedback is essential to reinforcement learning. Using the hit-and-test strategy, the AI component evaluates its environment before acting. It gains knowledge from previous mistakes and enhances its performance. Every successful action is rewarded, and every mistake is punished. Rewarding positive actions as much as possible is the goal of the reinforcement learning component.
Reinforcement learning lacks labeled data in contrast to supervised learning. Agents acquire knowledge through their experiences rather than through controlled learning. Think about video games. The activities of the reinforcement agent determine the state of the environment, which is determined by the game. Feedback for the agent might be offered through prizes and penalties. The overall game score will be impacted by this. Agents are anticipated to achieve a good rating.
Read More: Highlights the advantages and disadvantages of machine learning
Machine Learning evolution in Enterprise Resource Planning
The previous few years have seen considerable advancement in global ERP software. The next major development appears to be machine learning. ML, a subset of artificial intelligence, enables comprehension without the need for programming. Let's examine the various ways that ML changes ERP.
Look for the underlying issue
An ERP system with machine learning can use past data to help identify the underlying cause of a problem. A maintenance professional might be better equipped to identify any dangers or hazards, such as modifications done in Maintenance, Repair and Overhaul (MRO), and potential risks.
Targeted insights
Machine intelligence (ML) powered by ERP enables businesses to learn about their clients, processes, and workflows. These commercial insights not only improve the accuracy of observations but also allow them to focus on particular areas to comprehend them better. This can involve things like locating the source of a method's failure or discovering purchasing trends in specific places.
Prediction
Using ML in conjunction with ERP can help you create precise predictive analytics. ERPs are preferred by businesses because they allow for prediction.
Output and Production
ERP systems are more successful at boosting output capacity when machine learning is used. You can reduce waste and increase device efficiency. This will improve the efficiency of your business. ML can assist in locating the main source of issues in finished goods or rendered services.
Data Access
A great resource for providing a wealth of data is ERP. It might be challenging for many organizations to acquire this data and utilize it. One benefit of ML is its capacity for classifying data. You can gain insights into how to boost sales, manufacturing, or service from an ERP that makes use of machine learning. In achieving new objectives, these predictive data can be a big help. From a commercial standpoint, the data can be used to enhance marketing and sales.
ERP Advantages
Business resource planning software includes a wide range of full potentials that can be effectively turned into financial advantages. Let's look at the benefits of business systems right away.
Higher Management Performance
All aspects of business operations, including sales, human resources, inventory, supply, customer service, inventory, and manufacturing, are connected by ERP solutions, which serve as a central data hub. With computer technology, automated data handling is possible with ERP software.
Solutions for enterprise resource planning have some major benefits. They lessen paperwork and do away with the necessity for human data entry. Because of these advantages, there is less likelihood of human error, which can disrupt production. In addition, paper-based data can be translated into digital form. This makes it possible to allocate human resources directly.
Information is More Accurate and Readily Available
The other enterprise resource planning benefits stated in this article are affected by this basic benefit. Modern ERP systems make it possible to efficiently and centrally gather, process, and store data related to manufacturing, sales, supply, or customers. One data system can be utilized and maintained in place of numerous databases, each of which needs regular inspections and synchronization to eliminate redundant or outdated records.
By enhancing the quality of all internal data and facilitating employee access to it, performance at all levels of an organization may be optimized. The management of supply chains and inventories is the function that gains the most from the introduction of ERP.
Better Coordination
Collaboration across departments and branches of an organization is improved through a unified system that contains all business-related information. ERP software increases efficiency by cutting down on internal communication lag time. This is especially advantageous for businesses that have a network of affiliates spread across several states or continents.
Precise Planning
The majority of business data is readily available, making it simpler to assess and formulate plans. With the help of the combined data, management personnel and business analysts at the organization can create new projects or enhance those that already exist more effectively.
Reports Enhanced
All forms of reports, including financial, inventory, customer behavior, technical maintenance, and others, can be submitted quickly and easily with the help of ERP software.
Flexibility and Scalability
The benefit of modularity, which enables scaling up and down to accommodate changing situations, is another help of ERP software. With the aid of this capability, resource planning software can quickly adjust to the requirements of any firm, regardless of size. The business could be significantly impacted by even minor modifications to the organization's structure and operations. ERP programmes must be adaptable. ERP systems are versatile enough to accommodate any development, such as a rise in production, an expansion of an inventory, or the addition of additional users, features, or services.
Cost-Effectiveness
By minimizing paperwork, streamlining daily duties, and, most significantly, eliminating repeated processes, ERP systems can lower administrative costs. Resource planning systems can also be utilized to speed up commercial activities, like stock and supply management and logistics, which involve numerous information requests and approvals.
It's Simpler to Access Management Systems
ERP systems can be implemented as web- or mobile-enhanced apps based on unique resource planning requirements. They are accessible from anywhere and may be utilized on mobile devices like smartphones, tablets, and other similar gadgets.
Enhanced Client Services
Customer relationship management is enhanced by contemporary ERP software. The collection of all customer and sales-related data is made possible by centralized data storage. This facilitates client communication and cuts down on time needed to reply to client requests.
Faster access to more precise client history data is one of the advantages. The accurate collection and analysis of customer behavior data might result in better plan optimizations or better forecasts of the demand that must be satisfied at the right moment.
Enhanced Market Competitiveness
The total of all the advantages mentioned above in terms of financial gains for businesses is this. ERP systems give a company the biggest edge over rivals and enable it to compete in the market. Some companies opt to continue with tried-and-true methods or can't afford ERP solutions. Others modernize their companies and profit from technology advancements.
Disadvantages of ERP
While there are many advantages to using ERP solutions, it's crucial to be aware of their drawbacks before putting them to use in your company. Owners of businesses can eliminate or render ineffective these drawbacks.
Licencing and Development at a High Cost
The development of ERP solutions can be both time- and money-consuming. To create a resource planning system that satisfies customer requirements, a sizable and knowledgeable team is needed. Due to the increasing demand for professional workers and their high hourly rates, a customized solution may be pricey. The implementation of pre-made ERP systems can be done more quickly, but license fees can be extremely expensive. Ready-made ERP systems are more expensive, require more time to customize, have greater maintenance costs, and might not meet business needs or company operations.
High Cost of Deployment and Maintenance
A warning sign is when the cost of an ERP system is high. Such systems need to be installed and maintained, which costs more money and demands more personnel. Pre-made solutions need to be updated frequently, which could add to the cost. The increased efficiency across the board of the company, however, will make these investments profitable in the long run.
There Must be Practice and Training
IT employees must possess additional expertise due to the complexity of ERP software. Understanding an ERP system's fundamentals and even more to become proficient in all of its features can take a lot of work. The need for new ERP system users to take more time to learn them than those who have been using them for years is a second issue. By providing ample training materials and manuals, you can facilitate the process.
It Has to Be Adjusted
ERP software implementation demands accurate and cautious adjusting for success. This entails thorough personalization and adjustment. All layers of the enterprise's organization must be accommodated by ready-to-use and custom solutions.
It Takes Time to Reach Your Full Potential
It takes time to reach your full ability, and as the disadvantages of EPR systems make clear, this is not the case. The deployment process includes some steps, including customization, initial data input, and staff training, all of which can take some time.
Slow Software Development
The process of integrating an ERP system into your company might take some time, particularly if your hardware is antiquated. Depending on how complicated the programme is, it is not uncommon for ERP installs to take between two and three years. If you want to deploy an ERP system, you must make preparations years in advance to prevent work interruptions. Although they install more quickly, cloud-based ERP systems still take time.
Slow Data Transfer
The first time you use the new ERP system, you must import your data. Depending on the industry, this can take a while. If you have more data, this process will take more time. Even the integration of digital data into a new system can take some time. You must ensure that no duplicates or lost data are created during the migration. It is simple to upload and arrange data with the help of several ERP solutions that can be used with existing data storage systems. The data is simple to add.
Conclusion
ERP is a piece of software that integrates, controls, and aids in the organization of corporate activities. If the organization's culture does not change and the company does not look at how it can, the ERP transformation will be challenging.
Notwithstanding all the challenges, AI-powered ERP is the way of the future. Technology has already begun to alter how businesses run and how plans are made. Organizations all over the world are adjusting to make the most of this technology to enhance customer service and advance their commercial objectives. Already, ERP systems are very effective. It has reached a new degree of automation and efficiency thanks to AI. By lowering the need to intervene, large amounts of data can be eliminated, and decision-making quality increased.